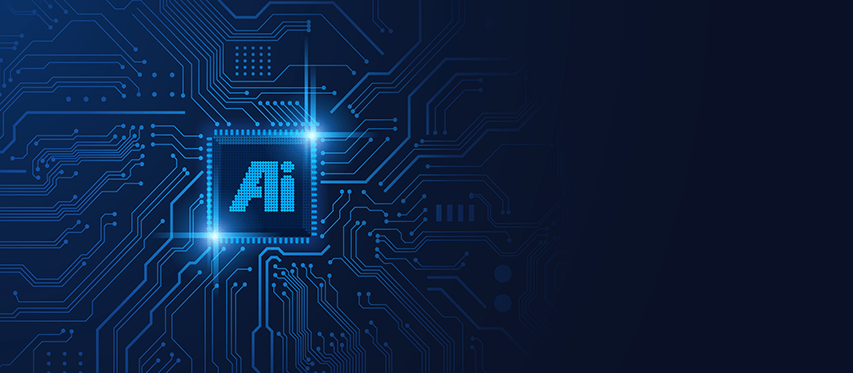
According to Ritu Jyoti, program vice president and research lead at International Data Corporation (IDC), "Early adopters [of artificial intelligence] report an improvement of almost 25 percent in customer experience, accelerated rates of innovation, higher competitiveness, higher margins, and better employee experience with the roll out of AI solutions.”
Hughes can be counted in that category. The company has been using years of network-related performance data to develop AI technologies designed to improve enterprise networking and IT operations, in what is known as “AIOps.” With an exploding number of connected users and devices and an endless flow of data, the possibilities for AI and ML application and innovation seem infinite.
Why Use AI/ML in Field Services Operations?
For years, Hughes technicians used mobile devices to take photos on the job during the installation process. That data was sent to the Hughes back office for random post-installation audits and analyses. Images might confirm if pole height was correct or if all connections were made properly. Yet with thousands of technicians, this manual process was time consuming.
The question for the team at Hughes, became, “How can we automate this process?”
“ML and AI are about building models to recognize patterns in existing data, and then using these (trained) models to identify situations and make predictions based on new or incoming data,” said Rupinder Bir, senior director, Hughes.
To automate the process for installation scenarios, the Hughes team applied ML and AI to stored installation images, training models to identify “good” versus “bad” images – those that illustrated both quality installs and errors, as well as denoted valid or invalid types of images.
The result is a mobile Installation Image classification system with real-time feedback capabilities. Hughes deployed the ML model on each technician’s mobile app and requires 10 photos be taken per installation. The app provides real-time feedback to the installer regarding whether the photo is being taken correctly or zooming in on the wrong spot. When positioned incorrectly, the app will not allow an image capture.
“When installing a dish for example, the installer takes pre- and post-install photos so we can record the connections they make and how they are installing for line-of-sight,” Mr. Bir explained. “If we have to perform a repair in the future and a new technician arrives on site, they have access to the images so they can see how the install was done previously.”
“The same ML model is also applied in the back office as a cross-check to review all of the images taken by our thousands of technicians throughout the day. It’ll flag where the install may not have been done correctly, because images must adhere to standards.” Logging the images also helps Hughes to collect installation metrics and promotes continuous improvement of the model itself.
More than 4.5 million images were processed in a 6-month period – roughly 15,000 images each day. A manual process would only have been able to complete 130,000 install records in that same timeframe, or 5% of the available data sets. Using ML and AI, Hughes increased audit efficiency by 95%.
When Technicians Speak, the Hughes App Listens
With the app deployed in the field, the Hughes team noticed that technicians did not submit detailed job-related information while on site because they dreaded typing on a mobile device. Consequently, the back office had access to limited data. To resolve the matter, the team integrated voice-to-text capabilities into the app using Natural Language Processing.
“We quickly saw a rise in submissions by eliminating the need to type and improving the technician’s ability to capture notes on-the-spot. That resulted in 50% more notes from the field, which are valuable for analyses and future troubleshooting,” he said. The enhancement also reduces data entry errors, like typos and misspellings, and saves technician time.
By 2025 the IDC study highlighted, 90% of enterprise apps will embed AI to make apps smarter and more dynamic. Clearly, Hughes took the lead on leveraging ML and AI to solve problems, fuel field service innovation, optimize operations, and improve the customer experience.